이번 시간 정리
XGBoost 모델 더 알아보기
- 트리 앙상블 중 성능이 좋은 알고리즘
- eXtreme Gradient Boosting를 줄여서 XGBoost라고 한다.
- 약한 학습기가 계속해서 업데이트를 하며 좋은 모델을 만들어 간다.
- 부스팅(앙상블) 기반의 알고리즘
- 캐글(글로벌 AI 경진대회)에서 뛰어난 성능을 보이면서 인기가 높아짐
1. XGBoost 불러오기
코드 실행
/opt/conda/lib/python3.9/site-packages/pkg_resources/__init__.py:122: PkgResourcesDeprecationWarning: 0.996-ko-0.9.2 is an invalid version and will not be supported in a future release
warnings.warn(
---------------------------------------------------------------------------
NameError Traceback (most recent call last)
/tmp/ipykernel_13/4205256983.py in <module>
2 from xgboost import XGBClassifier
3 model = XGBClassifier(random_state=0, use_label_encoder=False, eval_metric='logloss')
----> 4 model.fit(X_train, y_train)
5 pred = model.predict(X_test)
6 accuracy_score(y_test, pred)
NameError: name 'X_train' is not defined
2. 하이퍼 파라미터의 종류
- booster (기본값 gbtree) : 부스팅 알고리즘 (또는 dart, gblinear)
- objective (기본값 binary:logistic) : 이진분류 (다중분류: multi:softmax)
- max_depth (기본값 6) : 최대 한도 깊이
- learning_rate (기본값 0.1) : 학습률
- n_estimators (기본값 100) : 트리의 수
- subsample (기본값 1) : 훈련 샘플 개수의 비율
- colsample_bytree (기본값 1) : 특성 개수의 비율
- n_jobs (기본값 1) : 사용 코어 수 (-1: 모든 코어를 다 사용)
※ 아래 코드에서 하이퍼 파라미터 값을 직접 조절해 보세요.
코드 실행
---------------------------------------------------------------------------
NameError Traceback (most recent call last)
/tmp/ipykernel_13/4220802349.py in <module>
1 # xgboost 하이퍼파라미터
----> 2 model = XGBClassifier(random_state=0, use_label_encoder=False, eval_metric='logloss',
3 booster = 'gbtree',
4 objective = 'binary:logistic',
5 max_depth = 5,
NameError: name 'XGBClassifier' is not defined
코드 실행
---------------------------------------------------------------------------
NameError Traceback (most recent call last)
/tmp/ipykernel_13/3510154051.py in <module>
1 # 조기종료
----> 2 model = XGBClassifier(random_state=0, use_label_encoder=False, eval_metric='logloss',
3 learning_rate = 0.05,
4 n_estimators = 500)
5 eval_set = [(X_test, y_test)]
NameError: name 'XGBClassifier' is not defined
교차검증
일반적으로 모델을 학습시킬 때 데이터를 train set과 test set으로 나누어 train set을 가지고 학습을 수행합니다.
교차검증이란 여기서 train set을 다시 train set과 validation set으로 나누어 학습 중 검증과 수정을 수행하는 것을 의미합니다.
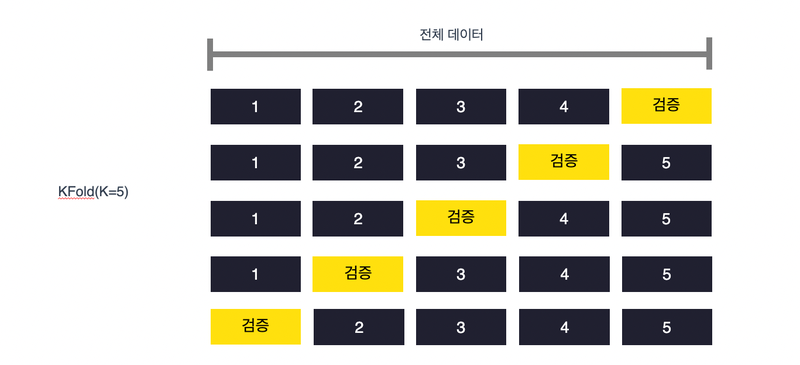
Kfold
- 일반적으로 사용되는 교차 검증 기법
코드 실행
---------------------------------------------------------------------------
NameError Traceback (most recent call last)
/tmp/ipykernel_13/250199126.py in <module>
4
5 kfold = KFold(n_splits=5)
----> 6 for train_idx, test_idx in kfold.split(X):
7 X_train, X_test = X.iloc[train_idx], X.iloc[test_idx]
8 y_train, y_test = y.iloc[train_idx], y.iloc[test_idx]
NameError: name 'X' is not defined
StratifiedKfold
- 불균형한 타겟 비율을 가진 데이터가 한쪽으로 치우치는 것을 방지
코드 실행
---------------------------------------------------------------------------
NameError Traceback (most recent call last)
/tmp/ipykernel_13/932449715.py in <module>
4
5 kfold = StratifiedKFold(n_splits=5)
----> 6 for train_idx, test_idx in kfold.split(X, y):
7 X_train, X_test = X.iloc[train_idx], X.iloc[test_idx]
8 y_train, y_test = y.iloc[train_idx], y.iloc[test_idx]
NameError: name 'X' is not defined
사이킷런 교차검증
- 사이킷런 내부 API를 통해 fit(학습) - predict(예측) - evaluation(평가)
코드 실행
---------------------------------------------------------------------------
NameError Traceback (most recent call last)
/tmp/ipykernel_13/1928490033.py in <module>
1 # 교차검증
2 from sklearn.model_selection import cross_val_score
----> 3 scores = cross_val_score(model, X, y, cv=3)
4 scores
NameError: name 'X' is not defined
코드 실행
---------------------------------------------------------------------------
NameError Traceback (most recent call last)
/tmp/ipykernel_13/3246143626.py in <module>
1 # 평균 점수
----> 2 scores.mean()
NameError: name 'scores' is not defined
코드 실행
---------------------------------------------------------------------------
NameError Traceback (most recent call last)
/tmp/ipykernel_13/3076507601.py in <module>
1 # 교차검증 Stratified Kfold
----> 2 kfold = StratifiedKFold(n_splits=5)
3 scores = cross_val_score(model, X, y, cv=kfold)
4 scores
NameError: name 'StratifiedKFold' is not defined
코드 실행
---------------------------------------------------------------------------
NameError Traceback (most recent call last)
/tmp/ipykernel_13/3246143626.py in <module>
1 # 평균 점수
----> 2 scores.mean()
NameError: name 'scores' is not defined
'언어 > 파이썬' 카테고리의 다른 글
머신러닝with파이썬6강(1)_선형회귀, 릿지회귀 (0) | 2024.03.13 |
---|---|
머신러닝with파이썬5강(3)_분류모델평가, 회귀모델평가 (1) | 2024.03.12 |
머신러닝with파이썬5강(1)_의사결정나무, 랜덤포레스트 (0) | 2024.03.10 |
머신러닝with파이썬4강(3)_사이킷런으로 머신러닝 진행하기, 사이킷런 공식문서 사이트 (0) | 2024.03.09 |
머신러닝with파이썬 4강(2)_데이터전처리: 범주형 데이터, 수치형 데이터 (0) | 2024.03.08 |